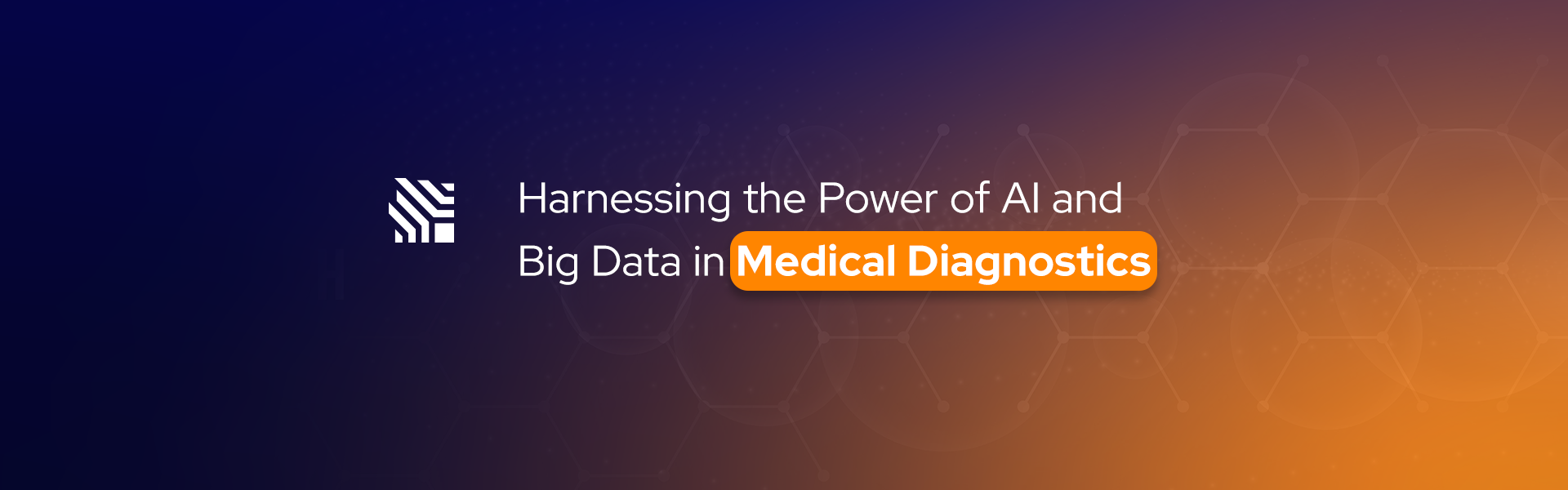
Harnessing the Power of AI and Big Data in Medical Diagnostics
The healthcare landscape has witnessed a paradigm shift in recent years, driven by the dual forces of Artificial Intelligence (AI) and Big Data. As we stand on the cusp of a new era, these technological marvels are redefining the boundaries of medical science, promising unprecedented advancements in patient care, diagnostics, and treatment modalities.
16 min read
In this Article:
- Evolution of Healthcare: The rise of Big Data and AI’s transformative role.
- Data Sources: Key contributors like patient records, wearables, and apps.
- AI in Diagnostics: Enhancing accuracy and a case study on breast cancer detection.
- AI Challenges: Addressing algorithmic bias and data security concerns.
- Role of Standards: Importance of IEC Standards in AI’s safe healthcare integration.
- Conclusion: The future potential and continuous evolution of AI in healthcare.
The Evolution of Healthcare with AI and Big Data
Historically, the medical field has always been data-rich, with patient records, clinical trials, and research studies generating vast amounts of information. However, the sheer volume and complexity of this data often made it challenging to derive actionable insights. Enter the age of digital transformation, where rapid technological advancements have catapulted healthcare into the realm of Big Data. From electronic health records (EHR) to advanced imaging modalities, the digital footprint of healthcare is expanding at an exponential rate.
Parallelly, the rise of AI has opened up new horizons in data analysis and interpretation. Gone are the days when medical decisions were solely based on empirical knowledge and individual experience. Today, AI-powered algorithms can sift through petabytes of data, identifying patterns and correlations that would be impossible for the human mind to discern. This synergy between AI and Big Data is not just a technological evolution; it’s a revolution that’s set to redefine the very fabric of healthcare.
The Potential of AI in Revolutionizing Medical Diagnostics
Diagnostics form the cornerstone of medical practice, guiding clinicians in their treatment decisions. With AI, the diagnostic process is undergoing a transformative change. Machine Learning (ML) algorithms, a subset of AI, are now capable of analyzing complex medical images, detecting anomalies with a precision that often surpasses human expertise. For instance, the early detection of diseases like cancer, which was once heavily reliant on the acumen of individual radiologists, is now being augmented by AI tools that can identify even the minutest of irregularities.
Moreover, Natural Language Processing (NLP), another AI facet, is making waves in the realm of clinical research. By analyzing vast repositories of medical literature, NLP algorithms can assist clinicians in staying abreast of the latest research findings, ensuring that patients receive care that’s in line with the most recent scientific evidence.
But perhaps the most significant promise of AI lies in its potential to personalize medical care. By analyzing a patient’s genetic makeup, lifestyle, and clinical history, AI can predict their susceptibility to various diseases, paving the way for preventive medicine. In essence, we are moving from a one-size-fits-all approach to a tailored healthcare model, where interventions are customized to the individual’s unique genetic and physiological profile.
The Rise of Big Data in Healthcare
Definition and Significance of Big Data in the Medical Field
Big Data in healthcare refers to the vast and complex datasets generated from various medical sources, which traditional data processing tools cannot efficiently handle. This data explosion is a direct result of the digital transformation that the healthcare industry has undergone in recent years. The significance of Big Data lies in its potential to provide deeper insights into patient health, disease patterns, treatment outcomes, and overall healthcare system performance. When harnessed effectively, it can lead to improved patient care, optimized treatment protocols, and a more holistic understanding of individual and community health.
Sources of Big Data
The sources of Big Data in healthcare are diverse and continually expanding:
Patient Records: Electronic Health Records (EHR) capture comprehensive patient data, from medical history to treatment plans, providing a rich source of information for analysis.
Telemonitoring: With the rise of telehealth, remote patient monitoring systems collect and transmit patient data in real-time, allowing for continuous health tracking and timely interventions.
Wearable Devices: Wearables, like smartwatches and fitness trackers, gather data on various health metrics, including heart rate, sleep patterns, and activity levels.
Healthcare Apps: Mobile health apps, designed for tracking nutrition, medication, mental health, and more, contribute to the data pool, offering insights into daily health habits and patient compliance.
The Potential of Big Data in Improving Healthcare Outcomes and Reducing Costs
Big Data’s potential in healthcare is multifaceted. By analyzing vast datasets, healthcare providers can identify trends, predict disease outbreaks, and tailor treatments to individual patient needs. Predictive analytics, derived from Big Data, can flag high-risk patients, enabling early interventions and reducing hospital readmissions. Furthermore, Big Data can streamline administrative processes, leading to cost savings. For instance, analyzing patient flow can optimize resource allocation, reducing waiting times and improving service delivery. Overall, Big Data promises a more efficient, cost-effective, and patient-centric healthcare system.
AI’s Role in Managing and Utilizing Big Data
The Limitations of Traditional Methods in Handling Vast Healthcare Data
Traditional data management tools are ill-equipped to handle the volume, variety, and velocity of healthcare data. Manual analysis is time-consuming and prone to errors, while conventional software lacks the sophistication to derive meaningful insights from complex datasets. Moreover, the dynamic nature of healthcare data, with continuous updates and real-time inputs, poses challenges for outdated systems.
Introduction of AI and Machine Learning for Efficient Data Management
AI and machine learning offer a solution to the challenges posed by Big Data. These technologies can process vast amounts of data at unprecedented speeds, identifying patterns and correlations that would be impossible for humans to discern. Machine learning algorithms, trained on historical data, can make predictions, classify data, and even assist in decision-making processes. For instance, AI can analyze patient records to predict disease risk or recommend personalized treatment plans.
Real-World Applications: Predictive Analytics, Disease Detection, and Clinical Decision-Making
The real-world applications of AI in managing and utilizing Big Data are vast:
Predictive Analytics: By analyzing patient data, AI can predict potential health risks, enabling preventive care and early interventions. For instance, AI can flag patients at risk of chronic diseases like diabetes or heart conditions, based on their medical history and lifestyle factors.
Disease Detection: AI-powered tools, especially in radiology, can analyze medical images to detect anomalies, aiding in the early diagnosis of diseases like cancer.
Clinical Decision-Making: AI can assist clinicians in making informed decisions by providing real-time data analysis, treatment recommendations, and even predicting patient responses to specific therapies.
The Accuracy and Precision of AI in Diagnostics
Minimizing Human Error in Diagnoses
One of the most compelling advantages of integrating AI into medical diagnostics is its potential to significantly reduce human error. Traditional diagnostic methods, while effective, are often subject to the limitations of human perception and interpretation. For instance, radiologists, despite their expertise, might overlook minute anomalies in medical images due to fatigue or the sheer volume of scans they review daily.
AI-powered diagnostic tools, trained on vast datasets, bring consistency and precision to the table. These algorithms can tirelessly analyze thousands of images, ensuring that even the most subtle irregularities are detected. By complementing human expertise with AI’s analytical prowess, the chances of misdiagnoses or oversight are drastically reduced, leading to more accurate and timely medical interventions.
Case Study: AI’s Impact on Breast Cancer Diagnostics
Breast cancer, one of the most prevalent cancers globally, often hinges on early detection for successful treatment outcomes. A study conducted by Beth Israel Deaconess Medical Centre (BIDMC) at Harvard Medical School showcased the transformative impact of machine learning on breast cancer diagnostics. The research revealed a staggering 85% reduction in human error rates in diagnosis when AI was employed.
The BIDMC team developed an advanced computational system designed to detect metastatic cells in lymph nodes. This AI-driven system boasted an impressive accuracy rate of 92%, highlighting its effectiveness in identifying and analyzing cancerous cells with unparalleled precision. Such advancements not only enhance the chances of survival for patients but also underscore the potential of AI in revolutionizing disease detection and management.
Addressing the Challenges: Algorithmic Bias and Data Security
The Potential Risks of Bias in AI Algorithms
While AI offers numerous benefits in healthcare, it’s not without its challenges. A significant concern is the potential for bias in AI algorithms. Since these algorithms are trained on real-world data, they can inadvertently inherit and perpetuate existing biases. For instance, if an AI system is predominantly trained on data from a specific demographic, its predictions and recommendations might not be as accurate for other demographic groups.
The Importance of Diverse and Representative Data
To combat algorithmic bias, it’s imperative to ensure that the training data is diverse and representative. This involves collecting data from varied sources, demographics, and geographies to create a holistic dataset. By doing so, AI systems can be more universally applicable, offering accurate predictions and recommendations across different patient groups.
Regulatory Compliance and Ensuring Patient Data Privacy
With the increasing digitization of patient records and the integration of AI tools, data security and privacy have become paramount. Ensuring the confidentiality of patient information is not just an ethical obligation but also a legal one. Regulatory frameworks, such as the Health Insurance Portability and Accountability Act (HIPAA), play a pivotal role in safeguarding patient data.
Moreover, as AI systems become more integrated into healthcare, there’s a growing need for robust cybersecurity measures. Protecting these systems from potential breaches is crucial to maintain patient trust and ensure the integrity of the diagnostic and treatment processes.
In conclusion, while AI and Big Data offer transformative potential in medical diagnostics, it’s essential to address the associated challenges head-on. By ensuring diverse training data, adhering to regulatory standards, and prioritizing data security, the healthcare industry can harness the full power of these technologies, leading to improved patient outcomes and a more efficient healthcare system.
The Importance of Standards in AI and Healthcare
The Role of IEC Standards in Ensuring the Safe Deployment of AI Technologies
As AI continues to make inroads into healthcare, ensuring its safe and effective deployment becomes paramount. The International Electrotechnical Commission (IEC) plays a pivotal role in this regard. IEC Standards act as a beacon, guiding the integration of AI technologies in healthcare settings. These standards are meticulously crafted to ensure that AI tools, regardless of their complexity, are used safely and efficiently.
IEC Standards address various facets of AI in healthcare, from the performance of medical equipment to the cybersecurity measures needed to protect patient data. For instance, IEC TR 60601-4-5:2021 provides detailed guidance on adapting IEC 62443 to the specific needs of the healthcare sector, ensuring robust cybersecurity for medical devices.
The Significance of International Standards for Medical Equipment and Software
International standards, like those developed by IEC, are not just technical benchmarks; they represent a global consensus on the best practices for medical equipment and software. These standards ensure that medical devices, irrespective of where they are manufactured or used, meet a consistent level of quality, safety, and efficacy.
Furthermore, with the healthcare industry becoming increasingly globalized, these international standards facilitate the interoperability of medical devices and systems across borders. This ensures that a patient, regardless of their location, receives consistent care quality, underpinned by universally accepted standards.
Conclusion
The Transformative Potential of AI and Big Data in Healthcare
The fusion of AI and Big Data in healthcare is nothing short of revolutionary. These technologies promise to redefine the very essence of patient care, offering more accurate diagnoses, personalized treatments, and a holistic approach to health and well-being. From early disease detection to predictive analytics, the possibilities are vast and transformative.
However, as with any technological advancement, the journey is not without its challenges. Algorithmic biases, data security concerns, and the need for diverse training data are just a few of the hurdles that the healthcare industry must navigate. But with the guidance of robust international standards and a commitment to continuous research and innovation, the future looks promising.
The Road Ahead: Continuous Evolution, Research, and Adherence to Standards
As we look to the future, it’s evident that the integration of AI and Big Data in healthcare is an ongoing journey. Continuous research, technological advancements, and adherence to international standards will be the guiding lights, ensuring that the full potential of these technologies is harnessed. The road ahead is one of collaboration, innovation, and a shared vision of a healthcare system that’s efficient, patient-centric, and driven by data-driven insights. The promise of AI and Big Data in healthcare is immense, and with concerted efforts, we can transform this promise into a reality.